- lazy val optimizedPlan = optimizer(analyzed)
-
- lazy val sparkPlan = planner(optimizedPlan).next()
SparkPlanner的apply方法,会返回一个Iterator[PhysicalPlan]。
SparkPlanner继承了SparkStrategies,SparkStrategies继承了QueryPlanner。
SparkStrategies包含了一系列特定的Strategies,这些Strategies是继承自QueryPlanner中定义的Strategy,它定义接受一个Logical Plan,生成一系列的Physical Plan
[java] view plain
copy
- @transient
- protected[sql] val planner = new SparkPlanner
-
- protected[sql] class SparkPlanner extends SparkStrategies {
- val sparkContext: SparkContext = self.sparkContext
-
- val sqlContext: SQLContext = self
-
- def numPartitions = self.numShufflePartitions
-
- val strategies: Seq[Strategy] =
- CommandStrategy(self) ::
- TakeOrdered ::
- PartialAggregation ::
- LeftSemiJoin ::
- HashJoin ::
- InMemoryScans ::
- ParquetOperations ::
- BasicOperators ::
- CartesianProduct ::
- BroadcastNestedLoopJoin :: Nil
- etc......
- }
QueryPlanner 是SparkPlanner的基类,定义了一系列的关键点,如Strategy,planLater和apply。
[java] view plain
copy
- abstract class QueryPlanner[PhysicalPlan <: TreeNode[PhysicalPlan]] {
-
- def strategies: Seq[Strategy]
-
-
- abstract protected class Strategy extends Logging {
- def apply(plan: LogicalPlan): Seq[PhysicalPlan]
- }
-
-
- protected def planLater(plan: LogicalPlan) = apply(plan).next()
-
- def apply(plan: LogicalPlan): Iterator[PhysicalPlan] = {
-
- val iter = strategies.view.flatMap(_(plan)).toIterator
- assert(iter.hasNext, s"No plan for $plan")
- iter
- }
- }
继承关系:

二、Spark Plan
Spark Plan是Catalyst里经过所有Strategies apply 的最终的物理执行计划的抽象类,它只是用来执行spark job的。
[java] view plain
copy
- lazy val executedPlan: SparkPlan = prepareForExecution(sparkPlan)
prepareForExecution其实是一个RuleExecutor[SparkPlan],当然这里的Rule就是SparkPlan了。
[java] view plain
copy
- @transient
- protected[sql] val prepareForExecution = new RuleExecutor[SparkPlan] {
- val batches =
- Batch("Add exchange", Once, AddExchange(self)) ::
- Batch("Prepare Expressions", Once, new BindReferences[SparkPlan]) :: Nil
- }
Spark Plan继承Query Plan[Spark Plan],里面定义的partition,requiredChildDistribution以及spark sql启动执行的execute方法。
[java] view plain
copy
- abstract class SparkPlan extends QueryPlan[SparkPlan] with Logging {
- self: Product =>
-
-
-
- def outputPartitioning: Partitioning = UnknownPartitioning(0)
-
- def requiredChildDistribution: Seq[Distribution] =
- Seq.fill(children.size)(UnspecifiedDistribution)
-
-
- def execute(): RDD[Row]
-
-
- def executeCollect(): Array[Row] = execute().map(_.copy()).collect()
-
- protected def buildRow(values: Seq[Any]): Row =
- new GenericRow(values.toArray)
- }
关于Spark Plan的继承关系,如图:
三、Strategies
Strategy,注意这里Strategy是在execution包下的,在SparkPlanner里定义了目前的几种策略:
LeftSemiJoin、HashJoin、PartialAggregation、BroadcastNestedLoopJoin、CartesianProduct、TakeOrdered、ParquetOperations、InMemoryScans、BasicOperators、CommandStrategy
3.1、LeftSemiJoin
Join分为好几种类型:
[java] view plain
copy
- case object Inner extends JoinType
- case object LeftOuter extends JoinType
- case object RightOuter extends JoinType
- case object FullOuter extends JoinType
- case object LeftSemi extends JoinType
如果Logical Plan里的Join是joinType为LeftSemi的话,就会执行这种策略,
这里ExtractEquiJoinKeys是一个pattern定义在patterns.scala里,主要是做模式匹配用的。
这里匹配只要是等值的join操作,都会封装为ExtractEquiJoinKeys对象,它会解析当前join,最后返回(joinType, rightKeys, leftKeys, condition, leftChild, rightChild)的格式。
最后返回一个execution.LeftSemiJoinHash这个Spark Plan,可见Spark Plan的类图继承关系图。
[java] view plain
copy
- object LeftSemiJoin extends Strategy with PredicateHelper {
- def apply(plan: LogicalPlan): Seq[SparkPlan] = plan match {
-
- case ExtractEquiJoinKeys(LeftSemi, leftKeys, rightKeys, condition, left, right) =>
- val semiJoin = execution.LeftSemiJoinHash(
- leftKeys, rightKeys, planLater(left), planLater(right))
- condition.map(Filter(_, semiJoin)).getOrElse(semiJoin) :: Nil
-
- case logical.Join(left, right, LeftSemi, condition) =>
- execution.LeftSemiJoinBNL(
- planLater(left), planLater(right), condition)(sqlContext) :: Nil
- case _ => Nil
- }
- }
3.2、HashJoin
HashJoin是我们最见的操作,innerJoin类型,里面提供了2种Spark Plan,BroadcastHashJoin 和 ShuffledHashJoin
BroadcastHashJoin的实现是一种广播变量的实现方法,如果设置了spark.sql.join.broadcastTables这个参数的表(表面逗号隔开)
就会用spark的Broadcast Variables方式先将一张表给查询出来,然后广播到各个机器中,相当于Hive中的map join。
ShuffledHashJoin是一种最传统的默认的join方式,会根据shuffle key进行shuffle的hash join。
[java] view plain
copy
- object HashJoin extends Strategy with PredicateHelper {
- private[this] def broadcastHashJoin(
- leftKeys: Seq[Expression],
- rightKeys: Seq[Expression],
- left: LogicalPlan,
- right: LogicalPlan,
- condition: Option[Expression],
- side: BuildSide) = {
- val broadcastHashJoin = execution.BroadcastHashJoin(
- leftKeys, rightKeys, side, planLater(left), planLater(right))(sqlContext)
- condition.map(Filter(_, broadcastHashJoin)).getOrElse(broadcastHashJoin) :: Nil
- }
-
- def broadcastTables: Seq[String] = sqlContext.joinBroadcastTables.split(",").toBuffer
-
- def apply(plan: LogicalPlan): Seq[SparkPlan] = plan match {
- case ExtractEquiJoinKeys(
- Inner,
- leftKeys,
- rightKeys,
- condition,
- left,
- right @ PhysicalOperation(_, _, b: BaseRelation))
- if broadcastTables.contains(b.tableName) =>
- broadcastHashJoin(leftKeys, rightKeys, left, right, condition, BuildRight)
-
- case ExtractEquiJoinKeys(
- Inner,
- leftKeys,
- rightKeys,
- condition,
- left @ PhysicalOperation(_, _, b: BaseRelation),
- right)
- if broadcastTables.contains(b.tableName) =>
- broadcastHashJoin(leftKeys, rightKeys, left, right, condition, BuildLeft)
-
- case ExtractEquiJoinKeys(Inner, leftKeys, rightKeys, condition, left, right) =>
- val hashJoin =
- execution.ShuffledHashJoin(
- leftKeys, rightKeys, BuildRight, planLater(left), planLater(right))
- condition.map(Filter(_, hashJoin)).getOrElse(hashJoin) :: Nil
-
- case _ => Nil
- }
- }
3.3、PartialAggregation
PartialAggregation是一个部分聚合的策略,即有些聚合操作可以在local里面完成的,就在local data里完成,而不必要的去shuffle所有的字段。
[java] view plain
copy
- object PartialAggregation extends Strategy {
- def apply(plan: LogicalPlan): Seq[SparkPlan] = plan match {
- case logical.Aggregate(groupingExpressions, aggregateExpressions, child) =>
-
- val allAggregates =
- aggregateExpressions.flatMap(_ collect { case a: AggregateExpression => a })
-
- val partialAggregates =
- aggregateExpressions.flatMap(_ collect { case p: PartialAggregate => p })
-
-
- if (allAggregates.size == partialAggregates.size) {
-
- val partialEvaluations: Map[Long, SplitEvaluation] =
- partialAggregates.map(a => (a.id, a.asPartial)).toMap
-
-
-
-
- val namedGroupingExpressions: Map[Expression, NamedExpression] = groupingExpressions.map {
- case n: NamedExpression => (n, n)
- case other => (other, Alias(other, "PartialGroup")())
- }.toMap
-
-
-
- val rewrittenAggregateExpressions = aggregateExpressions.map(_.transformUp {
- case e: Expression if partialEvaluations.contains(e.id) =>
- partialEvaluations(e.id).finalEvaluation
- case e: Expression if namedGroupingExpressions.contains(e) =>
- namedGroupingExpressions(e).toAttribute
- }).asInstanceOf[Seq[NamedExpression]]
-
- val partialComputation =
- (namedGroupingExpressions.values ++
- partialEvaluations.values.flatMap(_.partialEvaluations)).toSeq
-
-
- execution.Aggregate(
- partial = false,
- namedGroupingExpressions.values.map(_.toAttribute).toSeq,
- rewrittenAggregateExpressions,
- execution.Aggregate(
- partial = true,
- groupingExpressions,
- partialComputation,
- planLater(child))(sqlContext))(sqlContext) :: Nil
- } else {
- Nil
- }
- case _ => Nil
- }
- }
3.4、BroadcastNestedLoopJoin
BroadcastNestedLoopJoin是用于Left Outer Join, RightOuter, FullOuter这三种类型的join
而上述的Hash Join仅仅用于InnerJoin,这点要区分开来。
[java] view plain
copy
- object BroadcastNestedLoopJoin extends Strategy {
- def apply(plan: LogicalPlan): Seq[SparkPlan] = plan match {
- case logical.Join(left, right, joinType, condition) =>
- execution.BroadcastNestedLoopJoin(
- planLater(left), planLater(right), joinType, condition)(sqlContext) :: Nil
- case _ => Nil
- }
- }
部分代码;
[java] view plain
copy
- if (!matched && (joinType == LeftOuter || joinType == FullOuter)) {
- matchedRows += buildRow(streamedRow ++ Array.fill(right.output.size)(null))
- }
- }
- Iterator((matchedRows, includedBroadcastTuples))
- }
-
- val includedBroadcastTuples = streamedPlusMatches.map(_._2)
- val allIncludedBroadcastTuples =
- if (includedBroadcastTuples.count == 0) {
- new scala.collection.mutable.BitSet(broadcastedRelation.value.size)
- } else {
- streamedPlusMatches.map(_._2).reduce(_ ++ _)
- }
-
- val rightOuterMatches: Seq[Row] =
- if (joinType == RightOuter || joinType == FullOuter) {
- broadcastedRelation.value.zipWithIndex.filter {
- case (row, i) => !allIncludedBroadcastTuples.contains(i)
- }.map {
-
- case (row, _) => buildRow(Vector.fill(left.output.size)(null) ++ row)
- }
- } else {
- Vector()
- }
3.5、CartesianProduct
[java] view plain
copy
- 笛卡尔积的Join,有待过滤条件的Join。
- 主要是利用RDD的cartesian实现的。
- object CartesianProduct extends Strategy {
- def apply(plan: LogicalPlan): Seq[SparkPlan] = plan match {
- case logical.Join(left, right, _, None) =>
- execution.CartesianProduct(planLater(left), planLater(right)) :: Nil
- case logical.Join(left, right, Inner, Some(condition)) =>
- execution.Filter(condition,
- execution.CartesianProduct(planLater(left), planLater(right))) :: Nil
- case _ => Nil
- }
- }
3.6、TakeOrdered
TakeOrdered是用于Limit操作的,如果有Limit和Sort操作。
则返回一个TakeOrdered的Spark Plan。
主要也是利用RDD的takeOrdered方法来实现的排序后取TopN。
[java] view plain
copy
- object TakeOrdered extends Strategy {
- def apply(plan: LogicalPlan): Seq[SparkPlan] = plan match {
- case logical.Limit(IntegerLiteral(limit), logical.Sort(order, child)) =>
- execution.TakeOrdered(limit, order, planLater(child))(sqlContext) :: Nil
- case _ => Nil
- }
- }
3.7、ParquetOperations
支持ParquetOperations的读写,插入Table等。
[java] view plain
copy
- object ParquetOperations extends Strategy {
- def apply(plan: LogicalPlan): Seq[SparkPlan] = plan match {
-
- case logical.WriteToFile(path, child) =>
- val relation =
- ParquetRelation.create(path, child, sparkContext.hadoopConfiguration)
-
- InsertIntoParquetTable(relation, planLater(child), overwrite=false)(sqlContext) :: Nil
- case logical.InsertIntoTable(table: ParquetRelation, partition, child, overwrite) =>
- InsertIntoParquetTable(table, planLater(child), overwrite)(sqlContext) :: Nil
- case PhysicalOperation(projectList, filters: Seq[Expression], relation: ParquetRelation) =>
- val prunePushedDownFilters =
- if (sparkContext.conf.getBoolean(ParquetFilters.PARQUET_FILTER_PUSHDOWN_ENABLED, true)) {
- (filters: Seq[Expression]) => {
- filters.filter { filter =>
-
-
-
-
- val recordFilter = ParquetFilters.createFilter(filter)
- if (!recordFilter.isDefined) {
-
- true
- } else {
-
-
-
- !ParquetFilters.findExpression(recordFilter.get, filter).isDefined
- }
- }
- }
- } else {
- identity[Seq[Expression]] _
- }
- pruneFilterProject(
- projectList,
- filters,
- prunePushedDownFilters,
- ParquetTableScan(_, relation, filters)(sqlContext)) :: Nil
-
- case _ => Nil
- }
- }
3.8、InMemoryScans
InMemoryScans主要是对InMemoryRelation这个Logical Plan操作。
调用的其实是Spark Planner里的pruneFilterProject这个方法。
[java] view plain
copy
- object InMemoryScans extends Strategy {
- def apply(plan: LogicalPlan): Seq[SparkPlan] = plan match {
- case PhysicalOperation(projectList, filters, mem: InMemoryRelation) =>
- pruneFilterProject(
- projectList,
- filters,
- identity[Seq[Expression]],
- InMemoryColumnarTableScan(_, mem)) :: Nil
- case _ => Nil
- }
- }
3.9、BasicOperators
所有定义在org.apache.spark.sql.execution里的基本的Spark Plan,它们都在org.apache.spark.sql.execution包下basicOperators.scala内的
有Project、Filter、Sample、Union、Limit、TakeOrdered、Sort、ExistingRdd。
这些是基本元素,实现都相对简单,基本上都是RDD里的方法来实现的。
[java] view plain
copy
- object BasicOperators extends Strategy {
- def numPartitions = self.numPartitions
-
- def apply(plan: LogicalPlan): Seq[SparkPlan] = plan match {
- case logical.Distinct(child) =>
- execution.Aggregate(
- partial = false, child.output, child.output, planLater(child))(sqlContext) :: Nil
- case logical.Sort(sortExprs, child) =>
-
- execution.Sort(sortExprs, global = true, planLater(child)):: Nil
- case logical.SortPartitions(sortExprs, child) =>
-
-
- execution.Sort(sortExprs, global = false, planLater(child)) :: Nil
- case logical.Project(projectList, child) =>
- execution.Project(projectList, planLater(child)) :: Nil
- case logical.Filter(condition, child) =>
- execution.Filter(condition, planLater(child)) :: Nil
- case logical.Aggregate(group, agg, child) =>
- execution.Aggregate(partial = false, group, agg, planLater(child))(sqlContext) :: Nil
- case logical.Sample(fraction, withReplacement, seed, child) =>
- execution.Sample(fraction, withReplacement, seed, planLater(child)) :: Nil
- case logical.LocalRelation(output, data) =>
- val dataAsRdd =
- sparkContext.parallelize(data.map(r =>
- new GenericRow(r.productIterator.map(convertToCatalyst).toArray): Row))
- execution.ExistingRdd(output, dataAsRdd) :: Nil
- case logical.Limit(IntegerLiteral(limit), child) =>
- execution.Limit(limit, planLater(child))(sqlContext) :: Nil
- case Unions(unionChildren) =>
- execution.Union(unionChildren.map(planLater))(sqlContext) :: Nil
- case logical.Generate(generator, join, outer, _, child) =>
- execution.Generate(generator, join = join, outer = outer, planLater(child)) :: Nil
- case logical.NoRelation =>
- execution.ExistingRdd(Nil, singleRowRdd) :: Nil
- case logical.Repartition(expressions, child) =>
- execution.Exchange(HashPartitioning(expressions, numPartitions), planLater(child)) :: Nil
- case SparkLogicalPlan(existingPlan, _) => existingPlan :: Nil
- case _ => Nil
- }
- }
3.10 CommandStrategy
CommandStrategy是专门针对Command类型的Logical Plan
即set key = value 、 explain sql、 cache table xxx 这类操作
SetCommand主要实现方式是SparkContext的参数
ExplainCommand主要实现方式是利用executed Plan打印出tree string
CacheCommand主要实现方式SparkContext的cache table和uncache table
[java] view plain
copy
- case class CommandStrategy(context: SQLContext) extends Strategy {
- def apply(plan: LogicalPlan): Seq[SparkPlan] = plan match {
- case logical.SetCommand(key, value) =>
- Seq(execution.SetCommand(key, value, plan.output)(context))
- case logical.ExplainCommand(logicalPlan) =>
- Seq(execution.ExplainCommand(logicalPlan, plan.output)(context))
- case logical.CacheCommand(tableName, cache) =>
- Seq(execution.CacheCommand(tableName, cache)(context))
- case _ => Nil
- }
- }
四、Execution
Spark Plan的Execution方式均为调用其execute()方法生成RDD,除了简单的基本操作例如上面的basic operator实现比较简单,其它的实现都比较复杂,大致的实现我都在上面介绍了,本文就不详细讨论了。
五、总结
本文从介绍了Spark SQL的Catalyst框架的Physical plan以及其如何从Optimized Logical Plan转化为Spark Plan的过程,这个过程用到了很多的物理计划策略Strategies,每个Strategies最后还是在RuleExecutor里面被执行,最后生成一系列物理计划Executed Spark Plans。
Spark Plan是执行前最后一种计划,当生成executed spark plan后,就可以调用collect()方法来启动Spark Job来进行Spark SQL的真正执行了。
——EOF——
原创文章,转载请注明:
转载自:OopsOutOfMemory盛利的Blog,作者: OopsOutOfMemory
本文链接地址:http://blog.csdn.net/oopsoom/article/details/38235247
注:本文基于署名-非商业性使用-禁止演绎 2.5 中国大陆(CC BY-NC-ND 2.5 CN)协议,欢迎转载、转发和评论,但是请保留本文作者署名和文章链接。如若需要用于商业目的或者与授权方面的协商,请联系我。
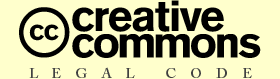
转自:http://blog.csdn.net/oopsoom/article/details/38235247
第六篇:Spark SQL Catalyst源码分析之Physical Plan
标签:字段 sam written 源码分析 scan str 卡尔 lex 执行计划